Il Gruppo BV TECH
Società
Persone
Milioni € di ricavi
%
R&D
“Il Progetto di Ricerca a garanzia della Sovranità e della leadership nel campo delle Tecnologie ICT e della Cybersecurity”
Raffaele Boccardo – CEO BV TECH
Soluzioni innovative di sicurezza informatica
SOC
Monitoraggio e analisi degli eventi di Cybersecurity.
SIEM
Raccolta e analisi delle informazioni di sicurezza.
Cyber Range
Simulazione di architetture di rete e di sicurezza.
NGFW
Next Generation Firewall modulare a elevate prestazioni.
NIDPS
Sonda di rete con funzionalità Deep Packet Inspection (DPI) e Intrusion Detection/Prevention System (IDS/IPS).
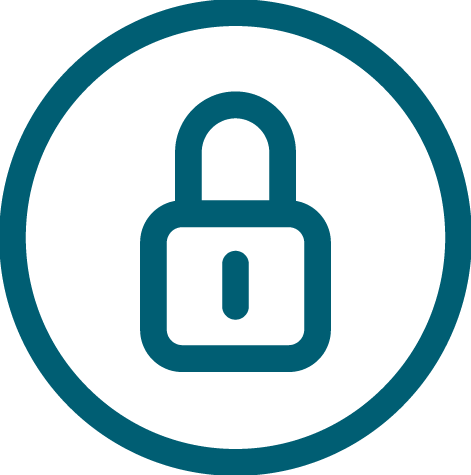
Asset Inventory
Sistema per Amministrazione, gestione allarmi, distribuzione software e verifica vulnerabilità delle applicazioni di sicurezza.
Situation Room
Piattaforma per una gestione avanzata delle informazioni di sicurezza e la creazione di una “Cyber Operational Picture” avanzata.
Passione e impegno per l’innovazione
Donne e Uomini con competenze interdisciplinari al servizio della ricostruzione dell’industria ICT per affrontare le sfide della Cybersecurity.
Un contesto lavorativo inclusivo per coltivare le potenzialità delle persone e favorirne la crescita e la partecipazione diretta alla costruzione diretta di valore.
Collaborazione consolidata con le migliori università e istituti di ricerca italiani e internazionali per la selezione, l’inserimento e la valorizzazione dei migliori talenti.
Percorsi di formazione e aggiornamento professionale continuo.
Partecipazione diretta ai grandi progetti di Trasformazione Digitale in Italia e nel Mondo.
News
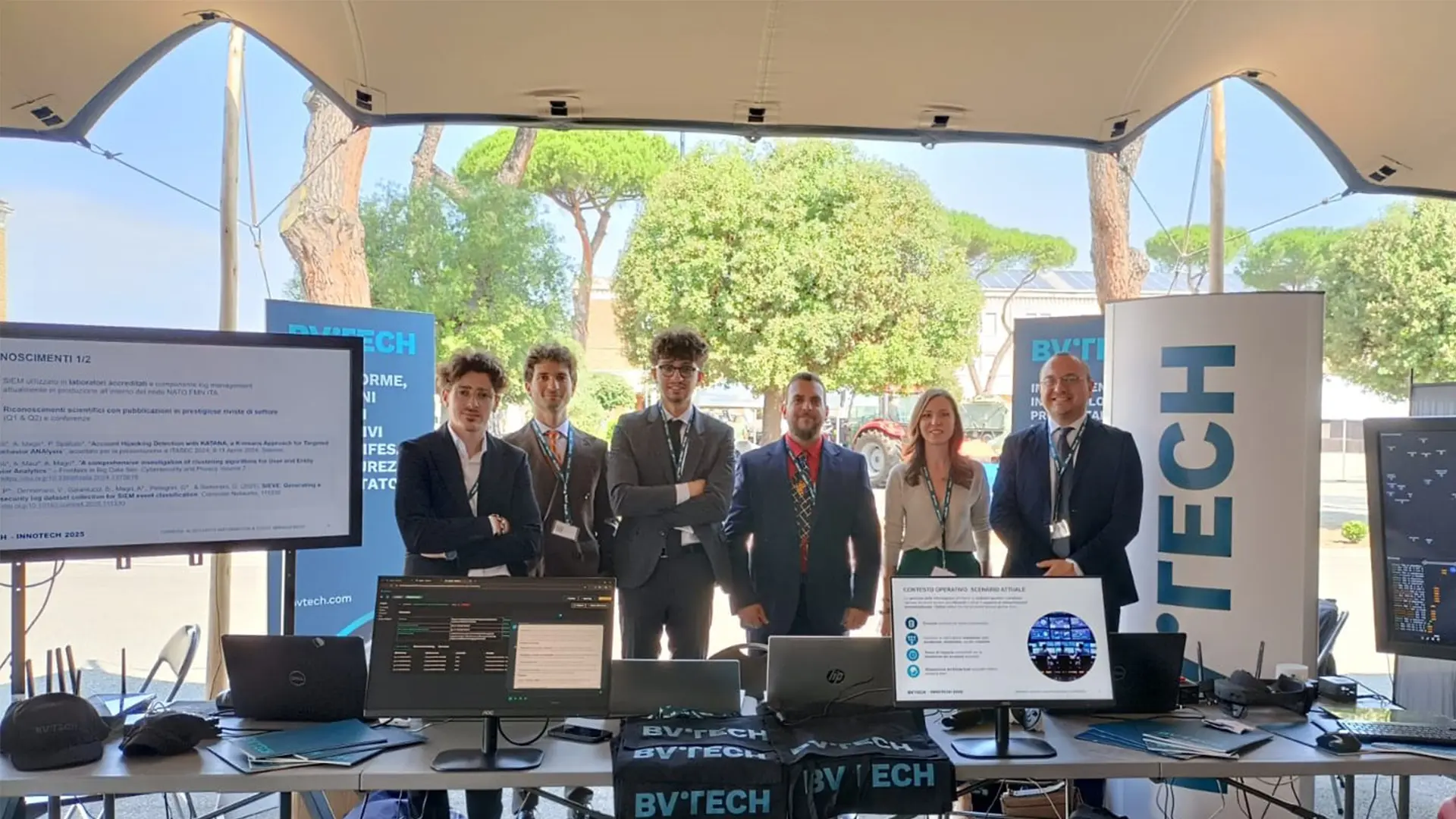
BV TECH a INNOTECH
25/06/2025
BV TECH ha partecipato all’evento “INNOTECH – Innovation & Technological C5I call: con i soldati, per i soldati”, organizzato dall’Esercito Italiano, presso la caserma “Perotti” della città Militare della Cecchignola a Roma.
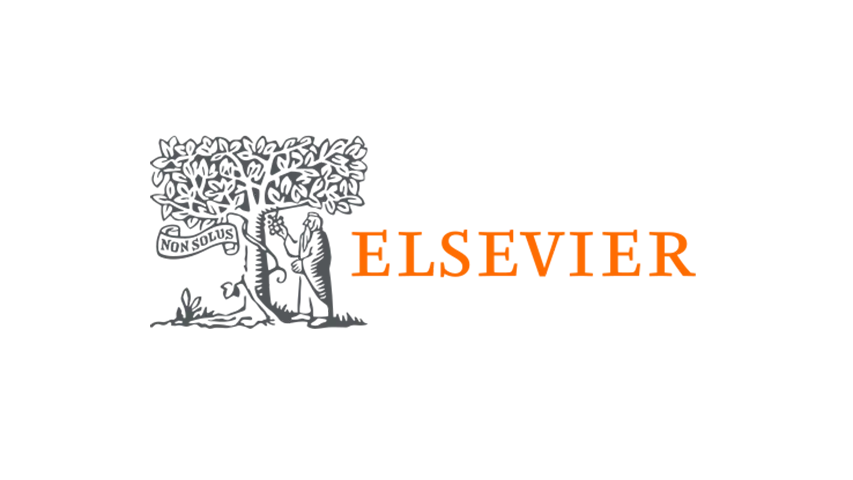
Il rapporto di ricerca “Human-Centered Interface Design For A DynamicCyber-Risk Group-Based Training Game” è stato accettato per lapubblicazione
05/06/2025
Il rapporto di ricerca è stato accettato per lapubblicazione.
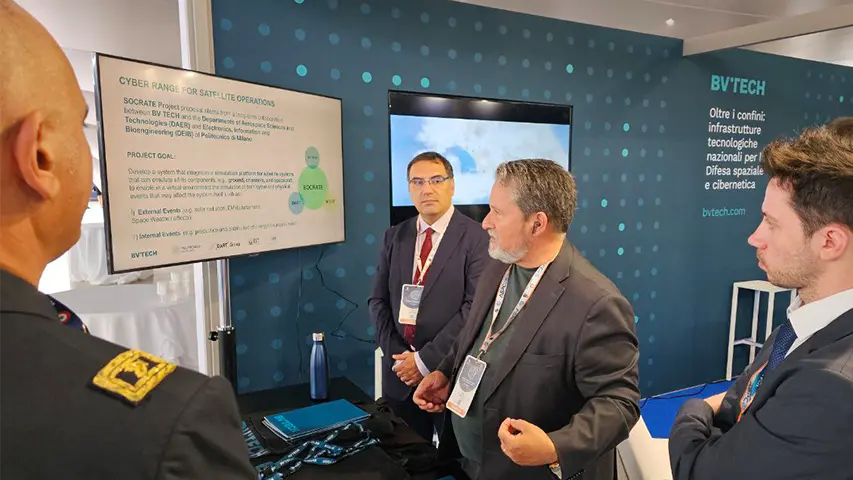
BV TECH Partecipa ad ASPC ’25
14/05/2025
Nell’ambito della Aerospace Power Conference 2025, BV TECH ha presentato presso il proprio stand i risultati di alcune delle attività di ricerca di interesse per il settore aerospaziale sia per applicazioni civili che in ambito militare.
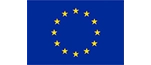
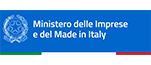
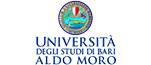
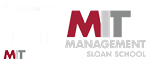
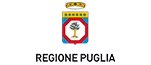
Progetto finanziato dal Fondo Europeo di Sviluppo Regionale Puglia POR Puglia 2014 – 2020 – Asse I – Obiettivo specifico 1a – Azione 1.1 (R&S), e con il supporto dell’Università di Bari e del Massachusetts Institute of Technology (MIT).